New Methods Help Fill Health Data Gaps while Protecting Privacy
Posted on by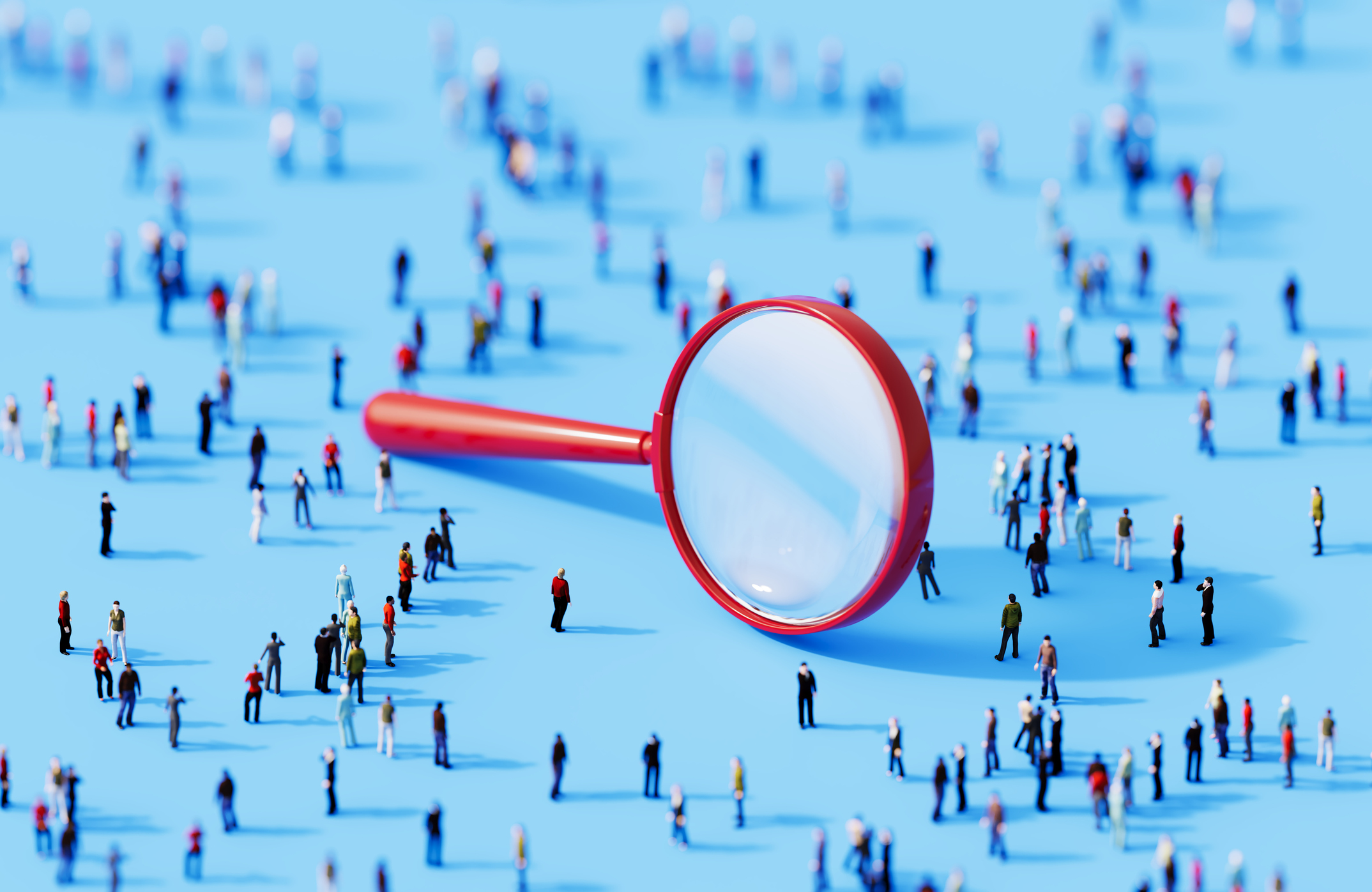
Innovative Statistical Strategies Provide “Synthetic” Data Developed from Survey Participant Responses
NCHS researchers compare methods for developing synthetic data for complex surveys, highlighting one that strikes the ideal balance between data usefulness and privacy protection.
When you fill out a health survey or provide personal information at a medical visit, you probably trust that your information stays confidential. But have you ever wondered exactly how your privacy is protected? At CDC’s National Center for Health Statistics (NCHS), statisticians explore new ways to protect your privacy while still providing high-quality data for research and public health.
Keeping data private is important, not just because it protects personal information but because public trust makes effective data collection possible. Without confidence that their information is secure, people might hesitate to respond to important surveys or share critical health details. Yet researchers, doctors, and public officials need accurate, useful data to identify health trends, develop effective treatments, and make informed policy decisions.
One way NCHS currently strives to ensure respondent privacy is to only allow access to certain data sets through restricted, controlled conditions. This approach, while providing confidence in data protections, may limit access for research because of cost and logistical issues.
One innovative solution to this challenge is called synthetic data. Through a careful design and modeling process, synthetic data looks and behaves like real data but doesn’t contain any actual personal information.
Recently, NCHS researchers tested different methods of developing synthetic data for complex surveys to find the best approach. Their goal? Striking the ideal balance between protecting individual privacy and making sure the synthetic data is accurate, reliable, and useful.
Let’s take a closer look at how synthetic data works and what NCHS researchers discovered.
The Story of Synthetic Data
One way to understand synthetic data is to think of it like a novel with realistic characters and scenarios that mirror everyday life.
In synthetic data sets, individual records are designed to reflect the patterns and characteristics found in real survey responses, but they do not correspond to actual people. One of the main challenges during the modeling process is balancing how accurately the data mirrors real life while still protecting privacy.
For example, public-use data sets often exclude information about distinctive traits, such as having a rare condition or living in a specific location, that could allow an individual to be identified. Yet without those details, it becomes harder to study how they might affect health.
“Standard modeling approaches, especially those that rely on random sampling from a synthesized population to protect privacy, can often fall short when applied to complex survey designs,” notes Guangyu Zhang, the lead author on the study.
These methods often ignore key survey design features, such as stratification, clustering, and weighting, which help make survey results more representative of the population. The resulting synthetic data may look realistic on the surface but can produce misleading results.
Preserving the structure of the original survey can help keep the data accurate and useful for analysis. But the closer synthetic data gets to the original, the greater the risk of revealing information about real people.
Finding the Balance
So, is it possible to increase access to high-quality complex survey data and ensure strong privacy protection?
To answer that question, NCHS researchers compared three different approaches for developing synthetic data, using widely available software and the Research and Development Survey (RANDS) as the original source.
In each approach, the team incorporated survey design features in different combinations, and applied both parametric and nonparametric modeling techniques to generate the synthetic data. These techniques are often used together to build and compare synthetic data sets.
Parametric methods, like linear or logistic regression, follow a fixed structure, like using a strict blueprint to build the new data. They rely on predefined assumptions about the data, such as how values are expected to cluster or vary.
Nonparametric methods, such as classification and regression trees (CART), are more flexible. They are more like building without a fixed plan and letting the structure emerge from the data itself.
The team evaluated the synthetic data sets to see how well they preserved the statistical properties of the original RANDS data and how effectively they protected individual privacy.
Approach | Results |
Approach 1 Combined (synthesized) all survey details, weights, and responses to the main survey questions in the new data |
Reduced data usefulness for the parametric method. The nonparametric method handled complex data structures better but posed slightly higher privacy risks compared with the parametric method. |
Approach 2 Used the survey details to guide, but only synthesized the weights and responses to the main survey questions in the new data |
Reduced data usefulness for the parametric method. The nonparametric method still handled complex data structures better. Both methods posed a higher risk of revealing personal information than Approach 1. |
Approach 3 Used the survey details and weights to guide but only synthesized responses to the main survey questions in the new data |
Improved data usefulness for the parametric method to the level of the nonparametric method. However, both parametric and nonparametric methods posed higher privacy risks than those of Approaches 1 and 2. |
Synthesizing the design information using the nonparametric method and responses to the main survey questions using the proper parametric method may stand out for striking the most effective balance. This approach included the original survey responses in the new data and the survey details or weights.
“Our approach directly synthesized survey data while maintaining the original data structure,” explains Zhang. “This method is straightforward to implement and very efficient.”
Both the parametric and nonparametric methods should be used for the best balance of providing useful data and strong privacy protection. The nonparametric method did a better job of preserving the complex structure of the original survey. It also posed a slightly higher risk of revealing personal information than the parametric method.
Overall, these methods can help statisticians and researchers effectively balance privacy and data usefulness when building synthetic data, depending on the data type.
“The research demonstrates that design information from complex surveys can be effectively synthesized using appropriate methods,” continues Zhang. “It serves as a valuable resource for federal agencies for data augmentation, testing and validation, and privacy protection.”
She also points out that the study uses readily available software packages (R synthpop, IVEware) that require minimal tuning or adaptation, making them useful for colleagues who generate synthetic data.
A Step Forward for Statistical Science
These findings provide practical guidance for statisticians and public health researchers who rely on synthetic data to support analysis while maintaining strict confidentiality protections.
While there’s more work to be done, the results demonstrate that with the right approach, synthetic data can serve as a tool to enable access to data for high-quality research while preserving respondent privacy.
Posted on by