Assessing Lifting Risk Factors Using Wearable Motion Sensors
Posted on by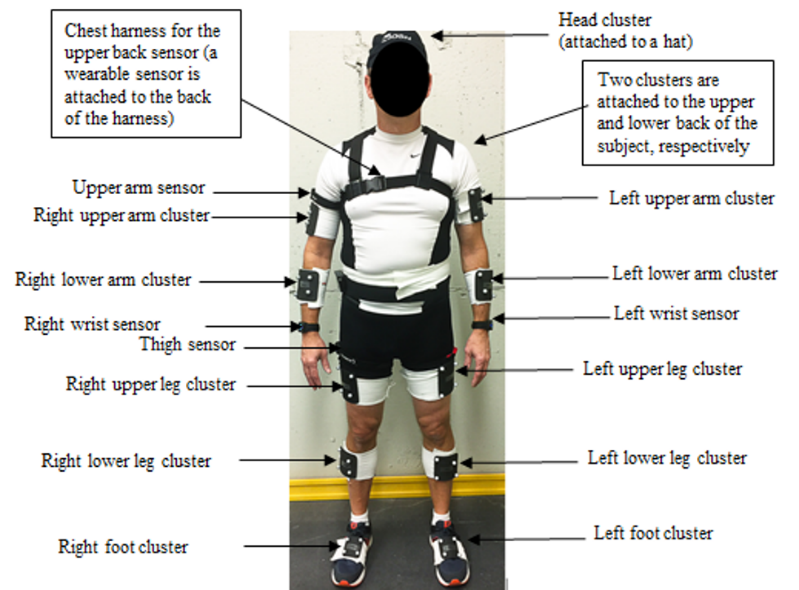
A combination of work-related physical risk factors such as awkward postures or heavy lifting may lead to an increased risk of developing low back issues. Those in the occupational safety and health field continue to conduct research to prevent workplace musculoskeletal injuries. Researchers have used industry settings, self-reports and observational methods to evaluate these injuries. However, these methods have limitations because of the subjective ratings of risk factors. Recent developments in sensor technology offer a great potential for improving traditional risk assessment methods. Inertial measurement unit- (IMU) based wearable technologies are becoming popular as a tool to track whole body postures for ergonomic risk assessments.[I]
IMU sensors (shown in figure) are small, lightweight, require little power and can monitor a worker’s postures over a long period. To make the most of wearable technologies for ergonomic risk assessments, several algorithms have been developed to measure body postural angles.[ii] [iii] [iv] Manual repetitive lifting has been identified as one of the main risk factors for musculoskeletal disorders.[v] [vi] However, algorithms for processing data from IMU sensors have not been developed for identifying the duration of a lifting task and other lifting risk factors.
The National Institute for Occupational Safety and Health (NIOSH) developed a popular lifting risk assessment method called “Revised NIOSH Lifting equation”.[vii] For this equation, vertical height (V) of the lifted object to the ground and the distance (H) between the lifted object and the body during a lifting task have been shown to be good risk predictors for low back pain (LBP).[viii] The identification of lifting tasks and their associated risk factors (V, H and lifting duration) using IMU sensors provides valuable risk information for interventions.
NIOSH researchers worked with the FocusMotion* and Kinetic* to develop algorithms that are capable of identifying lifting duration as well as V and H during lifting using only five IMU sensors. A typical research-grade system uses 13 to 17 sensors to capture whole body motions. The whole-body movement data provides information to estimate some LBP risk factors. However, these systems are expensive, cumbersome, and designed for research purposes. Each system costs at least $10,000-$35,000 each depending on the desired configuration. NIOSH performed tests to identify the smallest number of sensors that could be used to objectively assess LBP risk factors in variable working conditions.
The researchers collected body motion data on 10 individuals doing two-handed lifting tasks in various hand locations in a laboratory environment. The body motion was simultaneously recorded by five IMU sensors on the wrists, right upper arm, upper back, and right thigh and a research-grade motion capture system. The accuracy of the five-sensor system was assessed with data collected with the research-grade system. Researchers used 360 trials to test the algorithm’s capabilities. Two models were developed: a ratio model that uses only data from five sensors and a ratio and body segment length model that uses both sensor data and body segment length information. [ix] [x]
Main findings
- The algorithm was capable of measuring lifting duration within approximately one second of error.
- The algorithm performed well in measuring the trunk flexion angle (~2° error).
- The algorithm for the ratio model did not perform well in measuring V and H. The average errors for V and H were 33 cm and 6.5 cm, respectively.
- The algorithm for the ratio and body segment length model improved the accuracy of the V and H measurements to 14 and 2.2 cm, respectively.
Although the accuracy of the system is not quite desirable, this study indicates a potential for using IMU technology with a limited number of sensors to identify lifting-related risk factors. Costs for implementing such a system are expected to go down, as more effective algorithms may be discovered using machine learning approaches. Future research is needed to improve the estimations of various lifting risk factors using a limited number of sensors.
Are you using wearable technologies in your workplace? Please share your experiences in the comment section below.
Menekse S. Barim, PhD, AEP, is a Research Industrial Engineer in the NIOSH Division of Field Studies and Engineering.
Ming-Lun (Jack) Lu, PhD, CPE, is a Research Ergonomist in the NIOSH Division of Field Studies and Engineering and Manager of the NIOSH Musculoskeletal Health Cross-Sector Program.
*References to products, services, or companies does not constitute an endorsement by NIOSH or the U.S. government.
References
[i] Battini, D., Persona, A., & Sgarbossa, F. (2014). Innovative real-time system to integrate ergonomic evaluations into warehouse design and management. Computers & Industrial Engineering, 77, 1-10. Battini, D., Persona, A., & Sgarbossa, F. (2014). Innovative real-time system to integrate ergonomic evaluations into warehouse design and management. Computers & Industrial Engineering, 77, 1-10.
[ii] Seel, T., Raisch, J., Schauer, T. (2014). IMU-Based joint angle measurement for Gait Analysis, Sensors, 14 (4), 6891-6909.
[iii] Aoki, T., Feng-Shun Lin, J., Kulic, D., Venture, G. (2016). Segmentation of human upper body movement using multiple IMU sensors, 2016 38th Annual International Conference of the IEEE Engineering in Medicine and Biology Society (EMBC), 3163-3166.
[iv] Fang, Z., Yang, Z., Wang, R-B., Chen, S-Y. (2018). Inertial sensor-based knee angle estimation for gait analysis using the ant colony algorithm to find the optimal parameters for kalman filter, 2018 3rd International Conference on Automation, Mechanical and Electrical Engineering (AMEE 2018), 249-254.
[v] Bernard, B. P. (1997). A critical review of epidemiologic evidence for work-related musculoskeletal disorders of the neck, upper extremity, and low back. U.S. Department of Health and Human Services, Center for Disease Control and Prevention, National Institute for Occupational Health and Safety.
[vi] National Research Council (2001). Musculoskeletal Disorders and the workplace: low back and upper extremities. Washington, DC.
[vii] Waters, T.R., Putz-Anderson, V., Garg, A., (1994) Applications Manual for the Revised NIOSH Lifting Equation. DHHS (NIOSH) Pub. No. 94–110. U. S. Department of Health and Human Services, National Institute for Occupational Safety and Health, Cincinnati, OH.
[viii] Arjmand, N, Amini, M., Shirazi-Adl, A., Plamondon, A., Parnianpour, M. (2015). Revised NIOSH Lifting Equation may generate spine loads exceeding recommended limits, International Journal of Industrial Ergonomics, 47, 1-8.
[ix] Barim, M. S., Lu, M.-L., Feng, S., Hughes, G., Hayden, M., & Werren, D. (2019). Accuracy of An Algorithm Using Motion Data of Five Wearable IMU Sensors for Estimating Lifting Duration and Lifting Risk Factors. Proceedings of the Human Factors and Ergonomics Society Annual Meeting, 63(1), 1105–1111.
[x] Lu ML., Barim M.S., Feng S., Hughes G., Hayden M., Werren D. (2020) Development of a Wearable IMU System for Automatically Assessing Lifting Risk Factors. In: Duffy V. (eds) Digital Human Modeling and Applications in Health, Safety, Ergonomics and Risk Management. Posture, Motion and Health. HCII 2020. Lecture Notes in Computer Science, vol 12198. Springer, Cham
Posted on by