Artificial Intelligence Crowdsourcing Competition for Injury Surveillance
Posted on byIn 2018, NIOSH, the Bureau of Labor Statistics (BLS), and the Occupational Safety and Health Administration (OSHA) contracted the National Academies of Science (NAS) to conduct a consensus study on improving the cost-effectiveness and coordination of occupational safety and health (OSH) surveillance systems. NAS’s report recommended that the federal government use recent advancements in machine learning and artificial intelligence (AI) to automate the processing of data in OSH surveillance systems.
The main source of OSH information on fatal and non-fatal workplace incidents comes from the unstructured free-text “injury narratives” recorded in surveillance systems. For example, an employer may report an injury as “worker fell from the ladder after reaching out for a box.” For decades, humans have read these injury narratives to assign standardized codes using the U.S. Bureau of Labor Statistics’ (BLS) Occupational Injury and Illness Classification System (OIICS). Coding these injury narratives to analyze data is expensive, time consuming, and fraught with coding errors.
AI, namely machine learning text classification, offers a solution to this problem. If algorithms can be developed to “read” the injury narratives, data can be pulled from these surveillance systems in a fraction of the time of hand coding. Learn more in this related blog where AI coding was able to achieve in less than three hours what would have taken four-and-a-half years to manually code.
NIOSH developed an AI algorithm to apply OIICS codes based on injury narratives from a hospital emergency department surveillance system. However, the efficiency of this algorithm was not clear. To see if better coding algorithms could be developed, NIOSH turned to crowdsourcing.
While not unique to AI, crowdsourcing involves asking the crowd – or people in the public – with a variety of skill sets to provide their unique solution to a problem. The approach results in a large number of potential solutions that can be assessed to identify those that work best. Generally, the best crowd solutions are better than the original solution. In this case, NIOSH worked with two crowds – one internal to CDC and one external to CDC to propose better solutions to NIOSH’s initial coding algorithm.
Internal Crowdsourcing Competition
Before conducting an external competition, a team of seventeen researchers from NIOSH, the Centers for Disease Control and Prevention (CDC), BLS, OSHA, the Federal Emergency Management Administration, the Census Bureau, the National Institutes of Health, and the Consumer Products Safety Commission hosted a competition for staff at CDC. A total of nineteen employees competed to develop the best algorithm to code worker injury narratives. The team received nine algorithms, five of which outperformed the NIOSH baseline script, which had an accuracy of 81%. The internal crowdsourcing competition winning algorithm was 87% – a 6% improvement.
External Crowdsourcing Competition
In October 2019 NIOSH, together with National Aeronautics and Space Administration (NASA), hired a Tournament Lab vendor, Topcoder, to host the external crowdsourcing competition. This was the first-ever external crowdsourcing competition from CDC and NIOSH, which was partially funded through the CDC Innovation Fund Challenge. The competition accessed Topcoder’s global community of data science experts to develop a Natural Language Processing (NLP) algorithm to classify occupational work-related injury records according to OIICS.
Like the internal competition, the external competition was also a success! There were 961 submissions from 388 registrants representing over 26 countries (32% U.S., 21% India). Those participating self-identified as having degrees in: computer science and engineering, chemistry, computer engineering, computer science, data science, and economics to name a few. This competition produced 21% more registrants and 66% more submissions than the average Topcoder competition. The high-quality submissions achieved nearly 90% accuracy, which surpassed the 87% accuracy goal achieved during the internal competition.
And the winner is…
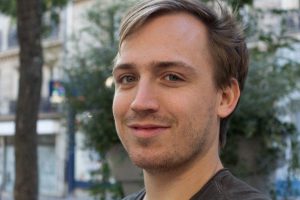
The 1st place winner was Raymond van Venetië who is a doctoral student in Numerical Mathematics at the University of Amsterdam. Second place was awarded to a senior data scientist at Sherbank AI lab in Russia; 3rd place was awarded to a developer and data scientist from China; 4th place was awarded to a biostatistician at the School of Medicine at Emory University in Atlanta, GA; and 5th place was awarded to a full stack engineer from Bangalore India.
What’s next?
The external competition and the resulting algorithm support improving efficiency and reducing costs associated with coding occupational safety and health surveillance data. Ultimately, we hope that the improved algorithm will contribute to greater worker safety and health. The NIOSH project team will work with the 1st prize winner’s script to make an easy-to-use web tool for public use. In the interim, the top 5 winning solutions are available on GitHub.
We want to hear from you!
Are you considering AI for data – or – have you used AI? What are your concerns or experiences using AI?
Sydney Webb, PhD, is a Health Communication Specialist in the NIOSH Division of Safety Research.
Carlos Siordia, PhD, is an Interdisciplinary Epidemiologist in the NIOSH Division of Safety Research.
Stephen Bertke, PhD, is a Research Statistician in the NIOSH Division of Field Studies and Engineering.
Diana Bartlett, MPH, MPP, is a Health Scientist in the CDC Office of Science.
Dan Reitz, is a Principal Public Sector Program Manager at Topcoder.
Posted on by