Colliding with Collider Bias: Implications for Precision Public Health
Posted on byA recent JAMA Guide to Statistics and Methods reviews how collider bias can lead to erroneous inference on causal relationships in clinical and epidemiological studies, potentially leading to incorrect clinical decision making and ineffective public health action.
What is Collider Bias?
Informed decision making in medicine and public health relies on valid evidence from clinical and epidemiological studies. Minimizing the risk of bias is critical for proper handling of research analyses and, ultimately, developing reliable interventions. One type of bias that can threaten the validity of study results is known as collider bias.
Collider bias occurs when an exposure and an outcome each influence a common third variable and that variable or collider is controlled for by study design or in the analysis (see figure below). Collider bias is different from confounding which occurs when an exposure and an outcome have a shared common cause that is not controlled for. Collider bias is often inadvertently introduced by controlling for a variable that occurs after the exposure. For example, collider bias can be introduced when study participants are systematically different from the population they represent at the beginning of a study or follow up over time. Low response rates or differential loss to follow-up in a study can lead to collider bias because the analysis is limited to a subgroup of the population.
Collider bias threatens the validity of study results by distorting relationships between exposures and outcomes. And it can work both ways. Collider bias can make associations appear real when there is not a true causal association in the general population. Collider bias can also dilute or hide underlying true causal associations.
An Example
Let’s take a recent example. In a 2020 retrospective cohort study of 4,480 patients diagnosed with COVID-19, researchers investigated whether use of angiotensin-converting enzyme inhibitor (ACEI) or angiotensin receptor blockers (ARBs) was associated with mortality or severe disease among patients diagnosed with COVID-19. The study found prior use of ACEI/ARBs was not significantly associated with mortality or severe disease among patients diagnosed with COVID-19.
The JAMA Guide to Statistics and Methods considers how collider bias applies to this study. ACEI/ARBs have been speculated to result in increased susceptibility for COVID-19. If this hypothesis were true, then collider bias may be a concern because the study is restricted to individuals with confirmed COVID-19. COVID-19 might represent a collider associated with drug treatment and mortality. Such inclusion criteria could generate a spurious negative association between the use of ACEI/ARBs and COVID-19 risk factors, which, through their association with mortality, can generate another spurious association between ACIE/ARBs and mortality. The authors of the study addressed the possibility of collider bias from sample selection by showing that ACEI/ARB was not associated with increased susceptibility to COVID-19, indicating that collider bias may not apply in this case.
This example illustrates the importance of scoping out study design and analytics and their potential for inducing collider bias and spurious associations. Illustrating the conceptual representation of a study using causal diagrams known as directed acyclic graphs (as shown in figure) may be helpful in identifying potential colliders.
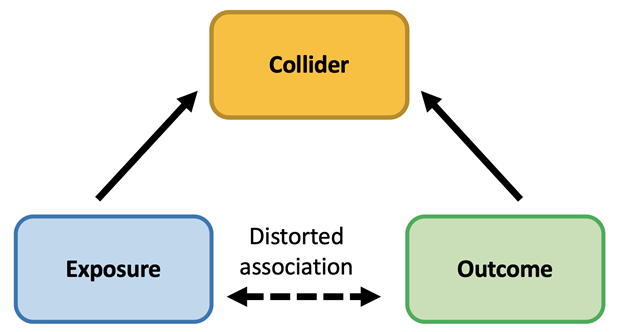
Impact of Collider Bias on Genomics and Precision Public Health
Large-scale genomic studies have uncovered thousands of statistical associations between genetic variants and health outcomes, transforming our understanding of the genetic determinants of human diseases. Nevertheless, study sample selection and attrition over time can bias associations between variables, generating potentially biased estimates for genetic associations. This bias can be magnified in the case of studying phenotypic associations with polygenic risk scores in large scale cohort studies of unrepresentative or highly selected populations, such as the UK biobank, and potentially in the newly launched All of Us Research Program cohort study.
As succinctly summarized by Munafo et al, “When polygenic scores that combine many genetic variants are used, association between the phenotype and participation will cause the score to be more strongly related to participation than each individual variant is. This, in turn, can potentially lead to serious bias. For this reason, studies using polygenic scores, genome-wide allelic scores and/or whole-genome genetic correlations are most at risk of producing biased and potentially misleading results where there is reason to believe the actual study sample is not representative of the intended study population.”
These observations highlight the value of representative cohorts such as birth cohorts, or population-based surveys such as the National Health and Nutrition Examination Survey (NHANES) where there is little or no selection into the cohort. In addition, the availability of data on all participants at recruitment into the study (e.g., DNA profiles, prevalence of risk factors and health outcomes) can allow us to assess the extent to which genetic and other factors predict subsequent participation. Without this extra information, large scale studies with unknown selection and attrition factors could provide biased or imprecise scientific inference for informing public health policy and medical decision-making.
We are interested to hear from our readers about examples of collider bias in genomics and precision public health and how they can be addressed in study design and analysis. Please submit your input here.
Posted on by